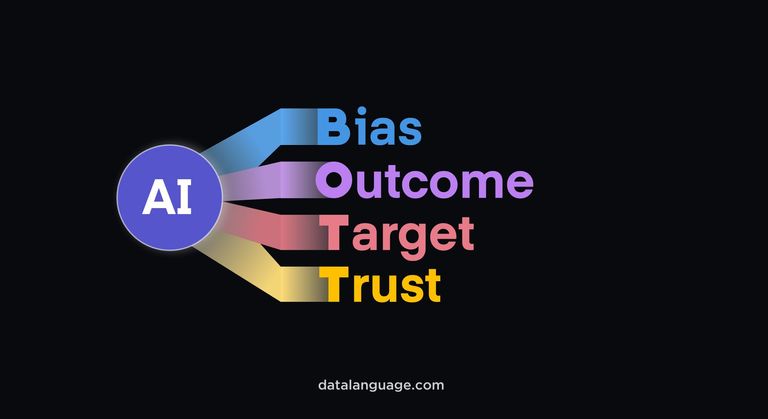
The BOTT Framework for Practical Data Ethics
As a data company, data ethics has always been important to us, but in the last few years, as Machine learning and AI have become front and centre as a significant part of digital transformation in the enterprise, data ethics has become a hot topic. It bears such a considerable weight in predictive analytics, not just in conveying trust to consumers of machine intelligence, but also in ensuring predictive models deliver genuine value, make unbiased predictions, and reflect the true state of the environment within which they live. When data ethics go wrong, it can go badly wrong, potentially bringing down an entire organisation, with substantial direct and indirect collateral damage, potentially even on national democracy and social cohesion - the Cambridge Analytica incident a case in point.
We all need to take it seriously.
In 2018 Data Language was privileged to have been accepted onto the Digital Catapult “Machine Intelligence Garage” programme. As well as supporting us with a terrific programme including compute resource, strategic help and industry engagement, Digital Catapult also took Data Language through their ethics programme. This was like a cold-shower, really waking us up to depth and breadth of data ethics. A realisation that data ethics is not just about data (technically), but about the way you approach your entire business.
With all this in mind, we were motivated to develop a data ethics framework that we could use internally at Data Language to assess all our predictive analytics projects, and that could guide us practically during implementation. We call this framework BOTT.
The BOTT Framework
BOTT (all good frameworks need a memorable acronym) stands for Bias, Outcome, Target and Trust. Through these four pillars, it allows our data science team to examine, and assess a predictive analytics model within its local and external ecosystem for quality, bias, and accuracy, and ensure it meets high standards of data ethics.
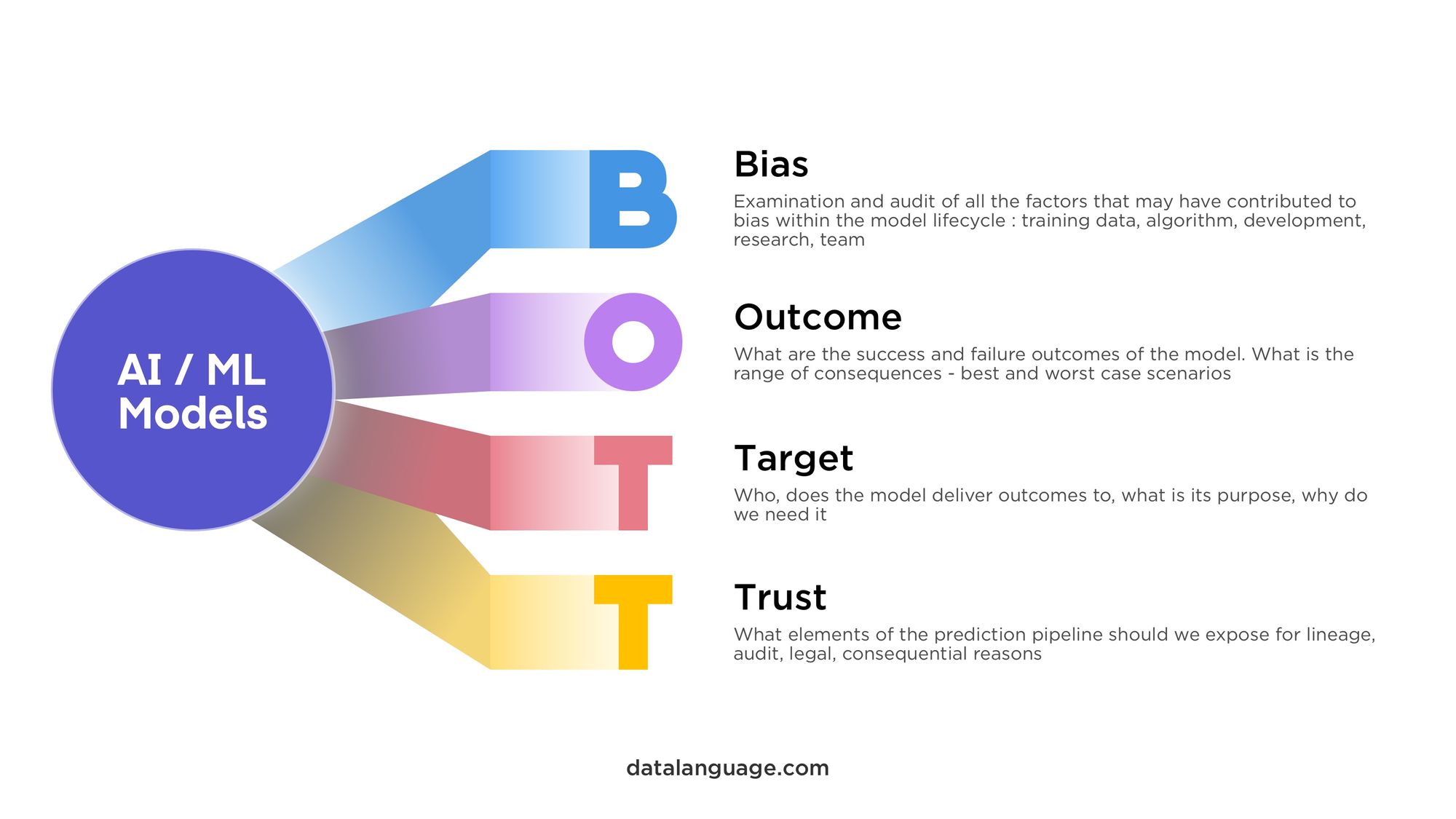
Bias
An analysis of potential bias that may be baked into the model during its life-cycle, either literally through data or code, or intangibly through for example, some conscious or subconscious human bias. We examine bias from the following perspectives :
Training data bias - is the training data diverse enough and comprehensive enough to accurately reflect the environment of the predictive model. What potential biases might skew predictive results either for good or bad outcomes - is there any chance these biases might exist in the training data we have used.
Algorithmic bias - is the model algorithm, its mathematical basis, or code potentially biased?
Real-time input data - if real-time (predict time) input data is involved in making some prediction, what might these data biases be, and how can we prevent them from impacting outputs.
Team bias - has or could conscious or unconscious bias of the model development team contribute to model bias, and have we mitigated against it. Often just an open and frank team discussion around bias (with respect to the project under consideration) is enough to flush out these sort of issues
Political, environmental, and social bias - similar to the team bias but looking at potential external factors through a similar lens - for both ideally the team needs to be very comfortable talking about potentially politically or socially sensitive topics in an open, friendly and non-defensive manner.
Output sampling - essentially QA - have you done effective output sampling and for quality assurance - does the risk of bias in the predictive model call for independent third party reviews in QA workflows, etc
Outcome
An analysis and survey of the full range of possible outcomes of the model:
Catalogue - construct a catalogue of the potential outcome scenarios
Impact - What does success and failure of the model mean :
- in the context of the target use-cases
- best and worst case scenario modelling
- model failure consequences, e.g. could somebody die if the model fails ?
Target
Analysis of who or what the model is targeted at and the context in which these users operate.
Users - Who are target users of the model - who or what is the direct consumer of the model ?
Purpose - What problem is the model solving?
Context: Is it part of an ensemble of models, is there a wider predictive ecosystem the model sits within - what impact does this model have within this context ?
Life span - What is the life expectancy of the model, and how does its environment change over time
Subversion - Can other users or consumers subvert the model for unintended/undesirable ends?
Catalogue - Construct a catalogue of potential unintended uses/consequences, and ascertain if those vulnerabilities can be designed out of the architecture?
Trust
Providing some visibility of the "behind the scenes" implementation can raise confidence in the models and foster a trust relationship between consumers and predictive services.
Explainable AI (XAI) is becoming a field in its own right, and an important part of machine learning and predictive analytics. Codable frameworks are emerging to aid explainability (for example Google Model Cards). This codability will be key in being able to compare and assess models through some normative evaluation mechanism.
Transparency - what should we be exposing of the lineage and decision making pipeline of the model prediction algorithm :
- training cycles / training samples
- outputs of intermediate predictions
- decision inputs/outputs
Impact - Why should these be exposed ? - perform an impact analysis of the possible effects of not exposing model lineage, with respect to legal, environmental, and model improvement consequences.