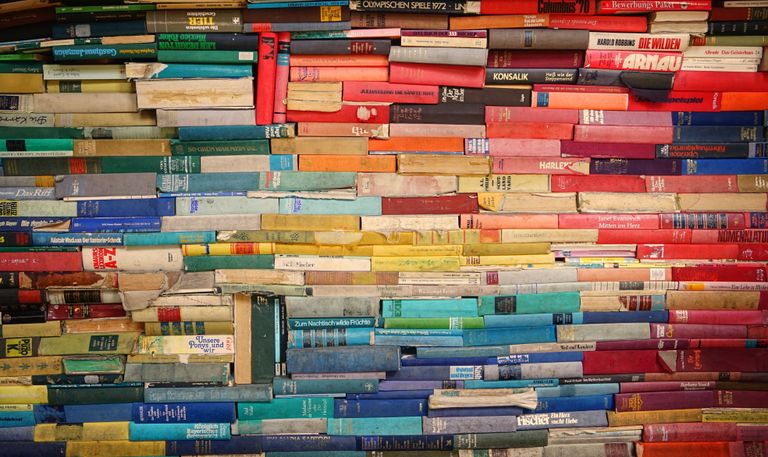
The surge of LLM models and their impact on Tagmatic
ChatGPT and LLMs in general have recently come into the public eye with a bang, and for good reasons: they represent a real opportunity to democratise access to machine intelligence for organisations and private individuals alike.
Beyond the current overhyped enthusiasm, and barring substantial obstacles that might be introduced at legislative level, we are about to witness substantial investment into the development and adoption of a plethora of plugins, platforms, APIs and services that leverage LLMs in one way or another.
At Data Language, we have simultaneously embraced the innovation opportunities as well as initiated an ongoing process for establishing whether LLMs might pose a disruptive risk to the business viability of our own products - Tagmatic in particular.
In their current form, LLMs appear to be unsuitable for business critical applications, particularly when deployed on tasks other than those focussed on generative capabilities.
Both OpenIA’s ChatGPT and Google’s Bard tend to (i) hallucinate, (ii) have architectural constraints with regard to the amount of context (ie memory) they can hold, and (iii) in general struggle to be constrained by external business logic. This may change in future, but given the very nature of how generative models work, these limitations are likely to persist to some degree.
Right now, some of these challenges may be mitigated by going down the “fine tuning” of the models - effectively specialising the base language models to behave in a given, business-specific fashion. This in turn implies, from a business perspective:
- Need to deploy resources (in-house or otherwise) to implement the fine tuning
- Need to source training data, and ensure it is of appropriate quality
- Need to source test data, necessary to evaluate the models’ output
- Depending on whether the base model to fine tune is remote (eg OpenAI’s, Google’s, ..) or private, there will be constraints to take into account, be legal, technical, or both.
- Businesses prepared to go down the fine tuning route, already have that option available. Open source transformers-based language models (eg BERT) have been around for years and can be trained to perform classification, sentiment analysis, question/answering, etc… Companies that have discarded that option due to the high Total Cost of Ownership have little reason to jump on the fine tuning of LLMs now.
- There is no guarantee that the new, fine tuned LLM would perform better and/or have a lower TCO of alternative solutions.
From a Tagmatic point of view, while obviously acknowledging the potential threat posed by a new breed of models, we remain convinced our product remains the best choice for organisations requiring a flexible, robust, enterprise-grade content classification solution:
- Our tests using IAB taxonomy showed ChatGPT to perform substantially worse than Tagmatic on a mere Precision/Recall level, even before hallucinations and inability to restrict tags to a custom set are taken into account.
- Tagmatic ability to perform real-time training and acknowledgement of new tags, is something unachievable with standard fine-tuning training, which are typically monolithic (the whole model needs to be retrained).
- Clients like NewsUK, currently see their Tagmatic model refreshing itself thousands of times daily!
- We have yet to see competitors score higher accuracy metrics. In fact, when compared to the likes of Google NLP the difference in output is stark.
- Tagmatic offers seamless, real-time, out of the box connectors to Data Graphs, making it the obvious choice for clients using Data Graphs as their knowledge repository. The sync’ing of the two systems is crucial for offering internal consistency of taxonomies.
- The Tagmatic analytics dashboard allows both business, editorial, and technical stakeholders to have a transparent view of the model’s performances, intercept trends, and isolate potential decays on a per-tag basis. Again, this is a critical component of onboarding AI at enterprise level that needs implementing one way or another: build-your-own is almost always the less convenient option
- Tagmatic comes with a set of options and configurations that can be tailored to suit the specific behaviour to be achieved. Again, this is not something widely available elsewhere.
- From an infosec perspective, Tagmatic has been designed to offer complete isolation between different clients’ instances, providing a completely ring-fenced environment.
- Last but not least, the team behind Tagmatic have a deep understanding of the publishing sector, having both been part of, and worked with, the industry for a number of years (BBC, Telegraph, NewsCorp, Springer, TV2, Financial Times, Euromoney, etc..)
In conclusion, we at Data Language are confident that Tagmatic is here to stay for the long run and offers fantastic value to its users: its unique architecture, high performances, and licensing model set it apart from other implementations (including LLM based) and make it an extremely strong choice in the text classification services landscape.
As always, we remain committed to our primary objective in all clients’ engagement: deliver the expert advice and solutions in their best interests. In this spirit, we do encourage our Tagmatic clients to explore novel technologies and services, compare results, and look beyond the current hype to convince themselves they remain at the forefront of innovation through the use of best in class products and services.