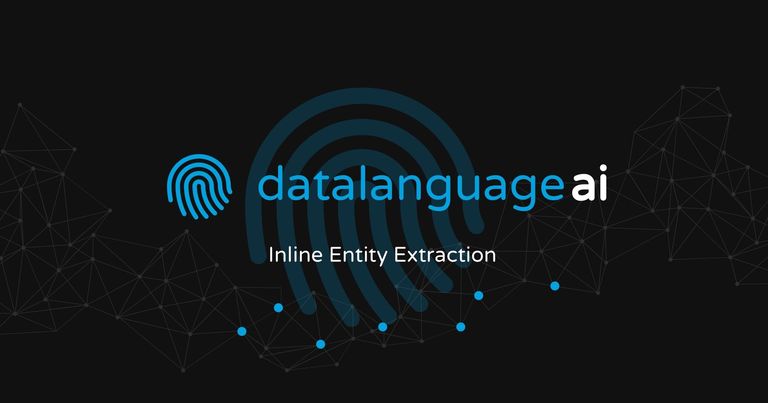
New Benchmarks from our Tagmatic Named Entity Recognition (NER) SaaS Product
We have recently completed some new "Inline Entity Extraction" benchmarks. This is a sophisticated "Named Entity Recognition" (NER) product that we launched as part of our Tagmatic product in 2020. It is optimized for Knowledge Graph platforms in high-value knowledge organizations. It identifies entities inline within text media and attributes rich metadata.
The Problem: Automated Tagging in Custom Domains
Our customers are high-value media publishers, knowledge organizations, and DaaS providers.
- A huge portion of their business asset value is locked in their unstructured mixed media assets.
- To liberate this value, accurate inline text classification of 100s of 1,000s of entities is required to enable structured connection with bespoke information architecture models and/or knowledge graphs.
- Generic off-the-shelf NLP SaaS solutions do not recognize these custom entities, so a mapping exercise is required, and is often a too low resolution to provide value.
- Many NLP solutions do not provide the rich, domain model-aware metadata, because they are not trained for the custom domain.
- Other custom NLP solutions require larger training sets and golden corpora that cover the entire entity set.
- "Build it yourself" becomes very expensive, with a large data scientist and engineering overheads, while organizations find themselves re-solving the same challenges that we have already solved.
- "Manage it yourself" has a surprisingly large data science and engineering overhead, to overcome production challenges that we have already solved.
Testing methodology
We trained our Inline Entity Extraction SaaS to understand around 180,000 entities from a custom domain. We then benchmarked it in a controlled environment where two teams of annotators were asked to annotate 500 documents:-
- The first team used the old-fashioned method of manually annotating a document.
- The second team moderated the output of the model that had already scanned the documents and picked up entities, only needing to confirm or reject pre-determined results.
Results: High F1 Score
Here’s how our Tagmatic Inline Entity Extraction SaaS performed:
- Precision: 0.94
- Recall: 0.90
- F1: 0.92
We have proven we can train a highly accurate model, specialized for a custom domain, that can cope with hundreds of thousands of entities with very high-performing metrics, using a golden corpus that covers only a small subset of the entities.
Achieving such high results on the classification of such a high number of classes is very rare for a model like this in the wild.
Results: Huge Workflow Efficiency Gains
The findings of this exercise were that the error rate of the team that used the Data Language AI model to pre-annotate the documents was substantially lower than the team that worked without the model.
- The team using "Tagmatic NER" demonstrated a 57% drop in error rate.
- The team using "Tagmatic NER" demonstrated a 53% increase in annotation coverage.
The measurable benefits were clearly efficiency and accuracy and added to the coverage of entities being substantially larger, it’s clear that using our model was a great success.
Should we keep Humans in the loop, or not?
We were also analyzing whether there is benefit in keeping humans in the loop and this result proved surprising. While it was clear that helping humans out in entity extractions tasks by pre-running the content through a model could significantly assist their operation, we actually found that the error rate of the model was actually slightly higher when humans were involved than when the results were totally automated. In this case, humans reviewing results actually makes things slightly worse!
There are some cases where perfect results are not mission-critical - e.g. upstream automated tagging to simplify downstream workflow, where "the machines do the heavy lifting". In these cases a certain amount of error is acceptable and a totally automated process will work.
In other cases, where errors are less acceptable, the confidence and evidence metadata in our SaaS can be used to allocate "less certain" predictions to SMEs and expert analysts for decisions and feedback loop optimizations.
Comparison to other Text Analytics NER Approaches
So what does our competition offer to compete with our results in the Named Entity Recognition (NER) space?
Well, it’s difficult to compare apples to apples in this scenario, as our competitors suffer from the same problem outlined above: Complex custom domains with small training corpora.
This is the exact advantage of our SaaS that these benchmarks emphasize.
To put these results into the context of standardized testing of NER performance elsewhere in the wider world and industry, one usually finds that most tests of F1 approach the value of 1, only where these models work by identifying a small handful of classes. Have a look - you will likely see "People, Places, Organizations, Events"... the usual suspects, and this problem itself has been solved daily for the last 12 years by almost every computer science student.
The Benefits of Tagmatic
The fact that our model can cope with a far greater number of classes than most other providers, and still see such impressive results, is a strong differentiator.
Another advantage of Tagmatic's capabilities is that they work out of the box at scale for your production platforms.
Our Inline Entity Extraction SaaS comes with all these benefits immediately:
- Ability to classify text content to 100s of 1,000s of entities in a custom domain.
- A rich API for interacting with the machine learning service metadata.
- Automatic management of machine learning model ‘fallout’.
- Self-optimisation of the machine learning models, on the fly.
- Capability to cope with an extremely high number of entities.
- The ability to scale up indefinitely.
- Production-ready engineering, out of the box.
All the engineering is done, and the security & maintenance are on us!
AI Relationship Extraction for Knowledge Graph Platforms
Later in 2022, we will launch a new commercial Knowledge Graph Relationship Extraction service as part of Text AI Services - early testing has impressive benchmarks for high-value knowledge organizations.
Find out more about Tagmatic.